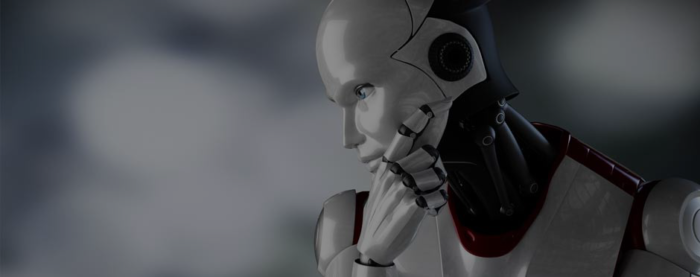
The article is aimed at any military officer or non-commissioned soldier with an interest in future technologies. No formal study in the field is required. The article is useful to appreciate the current pace in artificial intelligence designs and to inform realistic future military capabilities.
Reinforced Learning methods can be combined with data-driven specifications of behaviour to execute similar behaviour in the physical simulation of a robotic system. The following is a summary of several research articles drawn from Berkley Artificial Intelligence Research on current progress in robotic learning.
One method of robotic learning combined state-of-the-art techniques in computer vision and reinforcement learning, to simulate characters to learn a diverse repertoire of skills from video clips drawn from You Tube. Given a single monocular video of an actor performing some skill, such as a cartwheel or a backflip, characters were able to learn policies that reproduce skills in physics simulation, without requiring any manual pose annotations.
Another method employed a reinforcement learning paradigm to train an agent to solve an individual task with a manually designed reward. The reinforcement learning algorithms were used to learn multiple different tasks simultaneously, without additional human supervision. For an agent to acquire skills without human intervention, it must be able to set goals for itself, interact with the environment, and evaluate whether it has achieved its goals to improve its behaviour, all from raw observations such as images.
Imitation learning from a video of a human being can take a huge number of demonstrations hence a robot will struggle if there’s only one demonstration presented. Robots can be equipped with the ability to imitate by observing a human through meta-learning. Meta-learning involves the incorporation of prior experience rather than learning each skill completely from scratch. By incorporating prior experience, the robot should also be able to quickly learn to manipulate new objects while being invariant to shifts in domain, such as a person providing a demonstration, a varying background scene, or different viewpoint.
Computer vision, reinforcement learning through reward-based parameters and combining meta-learning with imitation learning allows robotic systems to observe and imitate behaviour within manually designated goals.
Within this area, Defence’s newest members will be exposed to the conflict spectrum and theories associated with its employment. While additional emphasis will be afforded to one’s own Service, consideration will be given to joint operations and planning processes. The topic will also include an exploration of current ADF operations and emerging technological impacts on future preparedness.
Personnel will be aware of cognitive biases associated with thinking fast and slow. They will also develop both their divergent (creative) and convergent (critical) thinking abilities through both formal learning and immersive application.
The ADF’s contribution to Australian National Power through shape, deter and respond activities will be understood in general terms. Tied with this awareness will be ADO roles and responsibilities, including civil-military relations. The importance of Defence’s contribution in this regard will be linked to a general sense of Australia’s strategic environment. Resource management fundamentals will help underpin responsible expenditure of Commonwealth funds.
All members of Defence will also need to be competent in military administration, ADF roles and structures, and undertake all initial mandatory training. This foundation will continue to develop in subsequent JPME levels and increasingly expand opportunites for Joint Stream (out-of-category) postings and roles. APS does not require core military skills.
Following on from their respective recruit or commissioning course, ADF personnel will commence their Initial Employment Training (IET). This period can include workplace experience and periods of on-the-job training.
Graduation from IET marks the formal transition from Level 1 to Level 2. It is at this point when they are considered effective in the workplace.
As Defence’s newest members, space is afforded to focus on leading self. This is a great opportunity to refine personal attributes that might not have been developed prior to enlisting. Positively influencing peers intersects with cultural alignment. Both bystander intervention and socialisation are important attributes in solidifying Defence’s cultural norms with those around us. For officers, leadership of others is introduced.
Being able to communicate effectively is critical to being a valued member of the team. JPME Level 1 introduces uniquely military forms of written and oral communication.
Newer members to Defence will focus primarily on the expectations of the organisation in terms of acceptable conduct. This will be dominated by appreciating the additional standards society holds them to, as well as military law (for ADF), and the moral codes of conduct. Defence’s core values serve as a foundation to this assimilation process. Increasing awareness of acceptable normative behaviour will be complemented with bystander intervention expectations. Ethics and values are closely linked to character development.
The nature of individual character is understood as a fundamental component of leadership. The development of individual character is accepted as relational in demonstrating respect and humility in interacting with others. The ability to build and foster positive relationships with others complements a resilient and moral identity as a junior leader in the Profession of Arms.
On joining Defence, members will develop an awareness of Defence culture and their own cultural norms while supporting an inclusive environment that recognises a wide variety of different cultural identities and backgrounds. This builds Defence cohesion and enables our success as representatives of a multicultural Australia when serving both domestically and overseas.
Defence members at this level will have a sound understanding of their own Service or domain warfare systems and capability. As they progress through Level 2, they will display a stronger understanding about the ADF’s holistic contribution to Joint Force warfighting domains. This will extend to the nature and theory of war, which has developed through an academic study of historical case studies and contemporary military issues. Importantly, such awareness will help inform their understanding of the impact of technology on current and future warfare. Awareness of gender perspectives on operations will contribute to their well-rounded application of the Profession of Arms.
By recognising the difference between complicated and complex problems, personnel at this level will ensure their problem-solving strategies and decision making are optimised. In the earlier stages, personnel will employ more transactional, linear thinking, decision support tools (such as the Joint Military Appreciation Process or variations) but they will increasingly apply alternate thinking strategies to seek more sophisticated options. Greater availability of human-machine teaming systems will progressively reduce our reliance on lower order cognitive activity and thus facilitate more nuanced considerations to complex problems.
Australia’s approach to the formulation of military strategy and its strategic environment (including international and geopolitical) will strengthen as members advance in this Level. Through both courses and experience, they will also acquire a stronger understanding of interagency strategies, policies and decision making processes.
As they progress through Level 2, Defence personnel will progressively develop awareness and then expertise beyond their initial employment category.
These broader systems and processes will expand to include one or more of the five key Joint Streams: Military Support, Operations, Capability Development and Delivery, Strategy Policy and Engagement, and Intelligence.
Within JPME Level 2, Defence personnel will become increasingly proficient in their initial employment category. Through both their formal courses (initial employment training and subsequent intermediate courses) and workplace experience, they will display competency in their contribution to military power. As they develop greater technical expertise, senior personnel at this level will become suitable for growing the next generation, by seeking instructional roles.
Moving into junior leadership roles, personnel at this level will begin leading small teams and be expected to lead leaders by the later stages of the level. Initial exploration of transformational leadership theories will evolve into more mature transformational approaches.
Both written and oral skills develop commensurate with greater leadership responsibilities. Personnel at this level are expected to be competent in communicating effectively across the range of Service writing documents and common delivery formats.
The focus of this level is to move from knowledge of Defence’s values towards a greater appreciation of ethical frameworks to help resolve moral dilemmas. Ethical understanding will emerge from a solid foundation in theories and justifiable decisions to challenging situations.
An evolving identity as a leader of character is supported by specific personal development. The application of character skills and qualities augments practical and professional experience in committed contexts. Experience also allows the development of greater self-awareness, consequent self-management, and ultimately more appropriate responses to situations across the spectrum of experience.
Aligned with their increasing leadership responsibilities, personnel will actively advocate a shared Defence ethos while appreciating and fostering our unique cultural differences as individuals and members of a Service or Group. We integrate growing self-awareness with active recognition of diversity. Our guidance of others enhances organisational cohesion and provides a solid foundation for working effectively with cultural difference
Those operating at this level will understand both internal Joint Force command relationships and international engagement with coalition partners. Their employment of operational art will consider instruments of national power and campaign design, while the relationship challenges between strategic, operational and tactical levels of war will be clearly understood. Continual professional development will keep Level 3 practitioners abreast of emerging trends and future warfare techniques. Expertise will be apparent in the planning requirements for force generation and the factors that shape the execution of joint operations. National and military strategic planning considerations for Volatile, Uncertain, Complex, Ambiguous and Novel (VUCAN) environments will mature. Personnel will be increasingly aware of the influence technology has on current and future warfare as well as whole-of-government response options.
Those operating at this level will be increasingly comfortable with systems thinking to perceive and influence complex adaptive systems. Various alternative thinking strategies, such as Design Thinking and Multi-Criteria Decision Making, will allow them to thrive in the challenging multi-domain environment.
Importantly, they will be able to translate complex strategic issues into tangible ideas for their subordinates.
This level is noted for its integration of civil-military functions and instruments of national power. This includes strategy and public policy development as well as contemporary operating environments and implications for military and security forces. Awareness of both Joint and multinational logistics will complement resource management systems of the Joint Force.
Expertise in one or more of the Joint Streams specific processes will continue to develop with staff and/or leadership responsibilities aligning with roles held.
Examples of the advanced knowledge base likely to be demonstrated include: One Defence Capability System (or its successor) and FIC management, Joint Force staff roles, advanced Human Resources systems and processes, Whole of Government and international engagement approaches, as well as legislative requirements associated with the relevant Joint Stream.
Through more senior category appointments and opportunities, those at Level 3 will display expertise within their specialist category employment. Completion of charge courses, deployments, exercises and pre-command courses, personnel will increasingly expand their specialist expertise to support joint effects.
Increasing leadership expectations will develop within their category’s specialist vocation.
At sub-unit command level, growing and developing other leaders is increasingly important. Based on more transformational leadership approaches, a mature sense of own leadership style will be apparent to others. Coaching and mentoring subordinates in all aspects of Professional Mastery will be expected. For officers and APS, both written and oral communication should now be at a polished staff officer level. Enlisted members will be expected to have a similarly high standard. However, the types and formats of communication will be often tailored to the position.
Resolving complex ethical dilemmas based on sound judgment and pluralist logic is expected at this level. A balanced understanding of major ethical approaches will assist in both moral leadership and ethical decision making.
Individuals have developed recognisable expertise as a character role model. The application of character skills, knowledge and experience is increasingly effective in complex, uncertain and ambiguous contexts. An ongoing commitment to being a leader of character is supported via self-activated opportunities to enhance versatility and experience. The addition of coaching and mentoring skills supports and encourages the development of the reflective self.
The ability to understand and influence foreign operations and environments while leading an inclusive team requires training in foundational and anthropological theories about human behaviour and cultures. At this level, stewards actively shape group identity, fostering individual skills and aligning team behaviours to effectively operate in a broad range of cultural contexts.
This level is recognised for its future Joint Force planning, development and sustainment challenges. Those operating in these positions will resolve military resource challenges and understand limitations of contracted support to operations. Operational artists will be adept at integrating strategic objectives to planning through the exploitation of emerging and non-traditional concepts for joint and multinational warfare. These skills will manifest in their ability to design and lead theatre strategies, campaigns and major operations. They will also be seeking opportunities to exploit technology’s influence on current and future warfare as well as its role in whole-of-government response options.
Cognitive performance will be enhanced through active seeking of diversity and a tolerance for ambiguity. Dealing with Defence-related wicked systems will be normal.
Problem-solving will increasingly accept increased risk to reflect the greater depth of wisdom and confidence in one’s judgments. This level is also noted for strategic thinking, visioning and championing a learning culture.
Level 4 leaders will be strong advocates of divergent thinking in their subordinates (when appropriate), to ensure Defence continues to improve systems and processes.
Those operating at this level have a very clear understanding of the impacts on national Defence policy and strategy. They will positively influence military capability development to enhance relevant instruments of national power. A strong awareness of contemporary global affairs and trends, combined with a deep understanding of international relations theories, will enhance their management of national military resources to support national security and foreign policy. Gender, peace and security impacts will continue to guide their strategic policy development.
Those operating at this level will be considered Generalists who possess a wide range of skills and expertise in multiple Joint Stream areas. With the exception of a select few specialists, these personnel will have actively sought out opportunities to broaden their versatility beyond their specialisation. Typical areas will include: strategic planning, civil-military relationships, campaign planning, strategic governance, business and financial acumen, One Defence Capability System leadership, strategic direction setting of emerging capabilities, policy leadership for future capability needs, and industry engagement for sustainment and growth.
At the enlisted level, Tier B and C warrant officers provide a vital link across the ADF, not only championing 75% of the ADF at the strategic table but also helping translate the top-down messaging. To do this, they must excel in understanding both worlds. Strategic level warrant officers also play a pivotal role in the command team relationship not only as advisors to decision-makers, but also in the strategic leadership of organisational culture, ongoing professional development, and the ideological underpinnings of the Profession of Arms throughout the ADF’s workforce
For those still in key roles within their primary category, there will be strong leadership expectations to ensure the delivery of capability, strategic advice on capabilities, limitations and future needs, as well as shaping the professional development system to grow future generations within the field.
In staff, command and post-command roles, authentic leadership will improve performance during change, transitions, crisis management and achieving results through diverse teams in VUCAN environments.
Exceptional Professional Mastery will transition to a mentoring and coaching role as will written and oral communication expertise. On a personal level, continued investment in peer networks will build future cooperation across Defence, Government and allies.
Complex ethical dilemmas will be resolved based on sound judgment and reasoned logic. This ability will come from a deep understanding across the spectrum of leading ethical approaches at a philosophical level.
Level 4 leaders will have a strong understanding of the difference between unit culture and climate in terms of ethical conduct. Importantly, they will actively mentor their subordinate leaders in the promotion of positive workplace climates.
Leaders of character are readily identifiable at this level and are credible and competent in generating a climate of trust. A personal commitment to exercising sound judgment and practical wisdom creates confidence across differing leadership contexts. Resilient behaviours and demonstrated composure create significant influence, shaping and realising organisational capacity and individual potential.
Mastery of key anthropological concepts generates deeper self-awareness and the resilience and agility needed to lead and act effectively in challenging and unfamiliar cross-cultural environments. These abilities will come from a highly developed capacity to reason and plan for cross-cultural challenges while building common objectives in a wide variety of cross-cultural settings, including operational, analytical, diplomatic and managerial contexts.
Senior officers and their equivalents will be comfortable in crisis leadership situations across all aspects of Defence. They will be highly effective when commanding Joint Task Forces or conducting influence operations. This performance will come from a deep understanding of Joint Force attributes, structures, capability requirements, emerging concepts and risks affecting military strategies and campaign development. Their strategic leadership abilities will extend to synthesising operational lessons learned across all Force elements including deployment, employment and sustainment. All of these areas will be enhanced by their astute awareness of political and resource implications in joint warfare strategy and planning.
Multi-agency, political and global wicked systems are an expected reality of Level 5 roles. Those operating at this level will have the cognitive agility to respond comfortably with these challenges by exploiting paradigm shifting approaches (such as nudge theory) to improve systems to a more advantageous state.
Senior leaders at this level will be expert in strategic communication and information impacts. They will comfortably implement grand strategy through future-focused policy formulation and employment of armed forces. Their expertise in national intelligence community and architecture will help them influence national security and foreign policy. The implications of contemporary challenges on national security strategies will be understood. This depth of understanding will be drawn from a strong awareness of national powers’ characteristics, capabilities and limitations combined with the civil-military relations necessary to deliver national power instruments.
Senior officers and SES band public servants at the highest echelons will be skilled in decision cycles and information/knowledge management systems. They will understand Government processes for capability acquisition and how to exploit technology enablers within enterprise systems.
As leaders of Force Element Groups (FEGs) or Joint Task Forces (JTFs), senior military officers will integrate emerging capabilities and technology to both maintain force-in-being deterrence and deliver operations to meet Government response options. In policy and advisory roles, they will exploit emerging capabilities and technologies when shaping military strategy, policy development and implementation. Industry engagement will enhance support of sovereign capability and Australia’s economic prosperity
A Service Warrant Officer is a unique appointment within each Service, one of influence and advice rather than Command. E10 Warrant Officers have strategic leadership responsibilities as Command advisors to Senior Leadership of their Service to provide critical insight to key decisions through an alternative lived experience and with support from Senior Enlisted Personnel to give a balanced, informed and analysed perspective from the workforce.
They also play a pivotal role in the organisation’s leadership, able to utilise their professional knowledge and experience to provide context and make the complex simple and the simple compelling. The unique appointment ensures the Service WO stays connected to contemporise issues for the workforce and represent those gaps and opportunities at the appropriate delegated level.
Service Warrant Officers deliberately steward, communicate and are genuinely dedicated to their Service; while always supporting strategic organisation culture and setting the conditions for long-term success.
Service Warrant Officers have a concurrent liability to foster relationships supporting Australia’s national power through networking and encouraging active engagement with counterparts to promote a commonality of purpose: – Friendship, Partnership and Leadership.
Senior officers and SES band public servants at the highest echelons will be skilled in decision cycles and information/knowledge management systems. They will understand Government processes for capability acquisition and how to exploit technology enablers within enterprise systems.
As leaders of Force Element Groups (FEGs) or Joint Task Forces (JTFs), senior military officers will integrate emerging capabilities and technology to both maintain force-in-being deterrence and deliver operations to meet Government response options. In policy and advisory roles, they will exploit emerging capabilities and technologies when shaping military strategy, policy development and implementation. Industry engagement will enhance support of sovereign capability and Australia’s economic prosperity
A Service Warrant Officer is a unique appointment within each Service, one of influence and advice rather than Command. E10 Warrant Officers have strategic leadership responsibilities as Command advisors to Senior Leadership of their Service to provide critical insight to key decisions through an alternative lived experience and with support from Senior Enlisted Personnel to give a balanced, informed and analysed perspective from the workforce.
They also play a pivotal role in the organisation’s leadership, able to utilise their professional knowledge and experience to provide context and make the complex simple and the simple compelling. The unique appointment ensures the Service WO stays connected to contemporise issues for the workforce and represent those gaps and opportunities at the appropriate delegated level.
Service Warrant Officers deliberately steward, communicate and are genuinely dedicated to their Service; while always supporting strategic organisation culture and setting the conditions for long-term success.
Service Warrant Officers have a concurrent liability to foster relationships supporting Australia’s national power through networking and encouraging active engagement with counterparts to promote a commonality of purpose: – Friendship, Partnership and Leadership.
Those operating at this level will be capitalising on and enhancing their peer networks across organisations, inter-agency and multinational fora. Within their own organisation, a strong, authentic leadership style will inspire excellence at all levels. They will also have robust enterprise, parliamentary, media and business acumen. These attributes will evolve from a blend of experience, advance study and self-reflection.
Stewarding the Profession of Arms at a national level will now transcend current cultural needs by anticipating emerging needs and setting the conditions for success in the future Force. Forward-leaning leadership in this area will ensure the organisation’s reputation as an ethical and virtuous national asset is widely recognised by the people of Australia.
A leader of character exemplar. Demonstrates meaningful commitment and creates a shared organisational understanding around the importance of character-based leadership. Provides tangible examples in personal conduct around ongoing self-development, developing others and setting an organisational climate conducive to lifelong character education and development.
Ambassadorship in this setting combines a full recognition of one’s own cultural worldview with the assured ability to understand culturally complex contexts, project national interests in multinational coalitions, and engage with the full range of national and international partners and operational environments. Cultural expertise will enable the advancement of national interests and negotiate strategic outcomes for Defence while representing Defence as a fluent cross-cultural ambassador.
Technical Mastery
Please let us know if you have discovered an issue with the content on this page.