Introduction
Artificial Intelligence is growing more capable, and its far-reaching implications are becoming apparent. Programs such as ChatGPT are reshaping many domains of human activity at a pace that challenges institutions to keep up.
Militaries have taken notice of the potential of Artificial Intelligence (AI). Open-source information suggests that both Russian and Chinese militaries are developing planning processes that incorporate AI.[1] The details of these military planning processes are not publicly available, but this should not deter us from comparing the ADF planning process to existing AI planning processes. Doing this will allow inferences regarding how the Joint Military Appreciation Process (JMAP) might be improved through the integration of AI.
Australia could improve the JMAP by integrating AI in the JMAP. This essay examines whether such integration might be possible, concluding that it is. It compares the JMAP to the planning process inherent in the computer program AlphaGo, analysing the applicability of AI-enhanced JMAP to the ADF from an ethical, theoretical, and practical perspective. I chose AlphaGo, a program that plays the board game Go, as the comparative planning process for several reasons. AlphaGo represents a state-of-the-art application of AI. The information about AlphaGo is largely public, unlike military applications of AI. Finally, it is famous (at least in AI circles) because it may have exhibited an example of genuine creativity in planning.[2] My comparison examines how the JMAP and AlphaGo perform two essential planning functions: framing the operating environment and developing and evaluating possible solutions. I conclude that the JMAP could be made more applicable to the ADF by better integration of AI in planning.
Background
Interest in augmenting military planning and decision making with technology is not new. AI-enhanced planning is a logical next step in a long trend of computerisation. In 1963 the US Air Force commissioned a report on methods to augment human intellect. Engelbart defined augmenting human intellect as ‘Increasing the capability of man to approach a complex problem situation to gain comprehension to suit his particular needs and to derive solutions to problems’.[3] It specifically identified computers as a powerful tool to achieve this.[4] AI is the most recent development in this regard. This pursuit of enhanced intellectual performance is deliberate today, with ADF doctrine directing that ‘The ADF must enhance the effect of our people through greater use of artificial intelligence’.[5] Although definitions of AI abound, Defence has defined AI as ‘the broad class of techniques in which seemingly intelligent behaviour is demonstrated by machines.’[6]
AlphaGo is one example of such an AI that seeks to win at the ancient Chinese strategy board game of Go. To do this effectively, AlphaGo must understand the environment and develop a plan to act. Comparing how AlphaGo does this with how the JMAP seeks to solve military problems provides the grounds for comparison and my subsequent analysis.
Understanding the Environment
JMAP has a distinct approach to understanding the environment. The first step of JMAP is scoping and framing that produces ‘detailed descriptions of the observed system and the desired system’.[7] Various methods are used including creating diagrams that capture actors, relationships, functions and tensions within the system. Such a diagram is a model of the system. There is a limit to the fidelity of any model. A visual representation, for example, is limited to three dimensions, with additional dimensional features being at best represented by other cues such as colouration. The goal, in the face of such constraints, is producing a model that adequately represents a complex system to inform the planner. In order to accommodate such constraints, ‘Scoping and framing may involve the need to deconstruct an ill-structured and/or ill-defined situation into a structured and understandable problem set.’[8] An infamous example of such an attempt is a NATO PowerPoint slide that attempts to graphically illustrate the actors and dynamics of the Afghan conflict (Figure 1).[9]
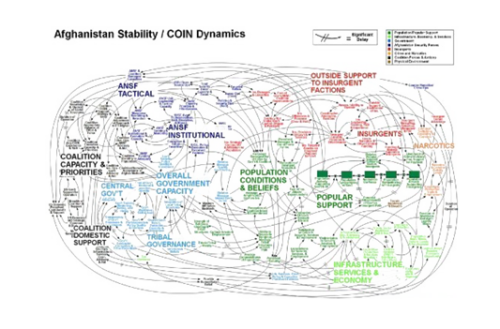
Figure 1 – Graphic model of the environment in Afghanistan illustrating the challenges of portraying complex systems or Ill-structured situations.
Scoping and framing is complemented by Joint Intelligence Preparation of the Operational Environment (JIPOE) which ‘constitutes a definition of the Operational Environment … and a description of environmental effects’.[10] This description informs the rules of the model developed in scoping.
Like JMAP, AlphaGo also develops a model of the environment, which in the game of Go is defined by the position of pieces on the board. It uses an approach like image classification and facial recognition software. The board, including the state of all pieces, is taken as a 19 by 19 image and layers in the neural network construct an increasingly abstract representation of the state of the board.[11] In doing so, this AlphaGo very literally ‘frames the environment’. Representation of the current state of the environment by a model is only part of understanding it. AlphaGo then uses a value network to evaluate a given board position, as well as the resulting board position that each move is likely to produce.[12] Its evaluation of each position is in terms of one simple metric: the probability of victory from that position. Although perhaps simplistic, the focus of this approach is entirely consistent with the first principle of war: selection and maintenance of the aim.
The approach to understanding the operational environment used in JMAP and by AI have similarities. Both seek to create a model of reality. In JMAP this model is instantiated in the biological brains of planners, aided by various cognitive artifacts such as diagrams, maps, and written text. There is also a model in AI, but it is encoded numerically in the multiple layers of AlphaGo’s neural network. Each model considers the environment as systems of nodes and links. There is nothing fundamentally incongruous about the way JMAP and AI describe the environment. Just because they use similar approaches, however, does not imply that JMAP and AI give similar results, ‘because an autonomous system may have different sensors and data sources than any of its human teammates, it may be operating on different contextual assumptions of the operational environment’.[13] Incorporating this diversity of perspective, human and non-human, can be expected to have the benefits broadly attributed to other forms of diversity. These benefits become apparent when the understanding of the environment is used to develop and evaluate courses of action.
Course of Action Development and Evaluation
JMAP course of action development and evaluation is the result of a dialogue between staff and commander. The ideas at the core of courses of action are often those of the commander, informed by a mix of experience, judgment, and intuition. As these processes occur in the mind of the commander, they are somewhat opaque. There are, however, externally visible elements of course-of-action development that can be analysed. Wargaming is one such possible source of novel ideas within the JMAP. In a contribution to the literature on the control of nuclear weapons, US nuclear strategist Schelling argued for the value of games in introducing novel ideas not otherwise available to planners. He observed, ‘one thing a person cannot do, no matter how rigorous his analysis or heroic his imagination, is to draw up a list of the things that would never occur to him!’[14] Wargaming can also be used to evaluate courses of action, similar to AlphaGo’s use of use of Monte Carlo simulation to make its assessments.[15]
AlphaGo considers courses of action iteratively, reassessing after each move to make the next legal move that has the highest possibility of resulting in victory. It does this by means of a policy network. This builds on the work of the value network in that: ‘The policy network takes a representation of the board position s as its input, passes it through many convolutional layers with parameters σ (SL [Supervised Learning] policy network) or ρ (RL [Reinforcement Learning] policy network), and outputs a probability distribution pσ(a|s) or pρ(a|s) over legal moves a, represented by a probability map over the board.’[16] As a result of the way it develops and compares plans, ‘AlphaGo has developed novel opening moves, including some that humans simply do not understand.’[17] AI used to develop courses of action can exhibit true creativity in this regard, made evident in 2016. During a victory over Korean Go champion Lee Sedol, AlphaGo made an extremely unexpected move (known widely as move 37). By placing a piece deep in Sedol’s area of the board, it defied conventional Go wisdom and baffled observers.[18] It so shook Sedol that he briefly left the room. This move changed the course of the game in AlphaGo’s favour, and its brilliance became apparent. Sedol was ultimately defeated by a creative move almost inconceivable to him, and to most humans. This provides a glimpse of how AI might enhance planning.
AlphaGo’s equivalent to course-of-action development and evaluation is similar to the JMAP in many respects. It is not a ‘brute force’ evaluation of all possible actions, but rather an informed development and assessment of distinct options. The result is that ‘AlphaGo evaluated thousands of times fewer positions than Deep Blue did in its chess match against Kasparov; compensating by selecting those positions more intelligently, using the policy network, and evaluating them more precisely, using the value network—an approach that is perhaps closer to how humans play.’[19] Similarly, wargaming does not consider endless number of courses of action but rather only those selected by the commander for development and perhaps only focusing on select aspects of those courses of action. The similarities with JMAP facilitate integration of AI.
AlphaGo and JMAP both make use of past examples to train for the future. The developers of AlphaGo detail various approaches taken to train different versions of the software that relied either on inputs of past human games of Go, or self-play against a simulated Go opponent. Training the algorithm on human-played games is analogous to the study of past campaigns: a pillar of professional military education. Interestingly, there seem to be parallel pitfalls to this approach as ‘The naive approach of predicting game outcomes from data consisting of complete games leads to overfitting.’[20] In other words: AlphaGo can fall into the trap, familiar to many militaries, of training to win the last war. A concerted effort was required to train the AI to make decisions that are optimal for a generalised future game/conflict.
Overall, the way in which AlphaGo understands the environment and develops and assesses courses of action resembles the JMAP but is sufficiently different that it might provide advantages. The JMAP in its current form fails to take full advantage of the differing strengths of human and AI available to solve military problems.
I next analyse AI’s applicability to the ADF planning to understand if these advantages might be seized upon. The applicability of AI to ADF planning needs to be considered from an ethical, theoretical, and practical perspective.
Ethical Applicability
Any analysis of AI in military planning must consider the ethical dimension to be applicable to the ADF. There is considerable ethical debate on AI in military decision making. It is particularly controversial to allow AI or autonomous systems to apply lethal force or make decisions that result in its application. Sparrow concludes that a human must always be directly responsible for the application of lethal force, and therefore lethal force cannot be directed by AI.[21] Simpson and Muller survey the debate, concluding that commanders can still be held accountable for decisions made by AI and it therefore remains permissible to use.[22] The inclusion of humans in an AI-enhanced JMAP further alleviates many concerns associated with unsupervised military decision making by AI.
The use of AI to generate courses of action leaves significant room for human control and oversight. The Monte Carlo approach used by AlphaGo could be guided by humans to produce plans more aligned with the desires of a commander, or to take into account moral considerations directed by a human planner. In their 1999 study, Myers and Lee use techniques beyond mere randomisation to generate qualitatively different plans through AI. Their approach was ‘rooted in the creation of biases, which focus the planner towards solutions with certain attributes’ such that ‘Users can optionally direct the planner into desired regions of the plan space by designating aspects of the metatheory that should be used for bias generation.”[23] This provides one possible form of human oversight on the making of lethal plans and decisions. (Note the term ‘bias’ is used above without any of its negative connotation, referring merely to the ability of humans to direct AI in particular way.)
The related issue of the legality of lethal autonomous weapon systems (LAWS) is subject to an ongoing debate at the United Nations, specifically the Convention on Prohibitions or Restrictions on the use of Certain Conventional Weapons which May Be Deemed to Be Excessively Injurious or to Have Indiscriminate Effects (abbreviated as the CCW). Australia’s 2020 submission to the CCW is generally favourable toward autonomous systems, saying, ‘Australia recognises the potential value and benefits that AI brings to military and civilian technologies.’[24] Australia argues for a broad definition of what construes human control of artificially intelligent systems. The submission concludes that the application of existing International Humanitarian Law is sufficient to address concerns about AI systems, leaving the door open to an AI-enhanced JMAP subject to extant review processes.[25] Australia would therefore seem to have no issue with the applicability of AI-enhanced planning to the ADF.
Theoretical Applicability
To analyse their applicability to the ADF, I consider the theoretical aspects of how JMAP and AI-enhanced planning might be applied by a military organisation. This requires understanding how such an ADF organisation acts, knows, and decides when it is engaged in planning. This is, at first, a sociological question. Building on a sociological understanding of a military organisation, cognitive science then provides a theoretical framework in which to understand the integration of human cognition and non-human elements.
Giddens argues that modern society is largely made up of expert systems, a category of entities that would encompass a military headquarters.[26] Expert systems have many properties, but how they relate to cognition is most important to the question at hand. Knorr-Cetina studies the behaviour of expert systems in science, showing how the conduct of certain activities shapes and changes an organisation. She identifies how High Energy Particle laboratories, in the conduct of experiments, ‘create a sort of distributed cognition, which also functions as a management mechanism: through this discourse, work becomes coordinated and self-organisation is made possible’.[27] Although her research focused on scientific laboratories as knowledge organisations, she conceded that the notion of epistemic cultures could be applied ‘to expert cultures outside science’.[28] I consider that a headquarters engaged in planning can exhibit distributed cognition, and exploring the notion of ‘distributed cognition’ further helps us understand how to consider the role of AI in planning.
The notion of distributed cognition allows me to assess the applicability of AI and the JMAP to the ADF. Vaesen summarises the theory by saying: ‘The basic idea behind distributed cognition (d-cog) is that cognition often distributes across individuals and/or epistemic aids such as instruments, diagrams, calculators, computers, and so forth.’[29] Hutchins famously illustrates distributed cognition with the example of a ship navigating into port.[30] After extensive study aboard US Navy ships, he came to conclude that the system of humans and instruments together direct the ship. This system achieved cognitive results outside any one human mind, and more than the sum of the individual cognition of each human in the process. Seeing AI in this manner reveals that it is merely another non-human element in a system that already integrates various cognitive artifacts. AI is as applicable to the JMAP as the other technologies (computers, matrices, visualisations, maps) that are already integrated in ADF planning. This understanding of a headquarters as a distributed cognitive system underlines the potential that integrating AI and human intelligence will produce emergent benefits that are not merely the sum of the headquarters’ digital and biological parts. Further, as military headquarters already distribute cognition between humans and artifacts, there is no theoretical obstacle to AI integration.
Practical Applicability
Practically, what would a more advantageous integration of AI in the JMAP look like? Would changes to the planning staff structure resemble the disappearance of clerical and typing pools with the advent of personal computers? Would there be a requirement for new staff functions to clean and manage data, or adapt and refine algorithms? The 2017 creation of an Algorithmic Warfare Team by the US Department of Defense offers some idea how such teams might add value to existing structures and functions in Defence.[31] In his 2019 article, Ryan observed how the ADF planning process ‘could be significantly enhanced and potentially sped up through the application of AI-extenders that could develop models of action, testing and comparing various activities against known and projected enemy capabilities—and then comparing different courses of action for their capacity to achieve higher-level outcomes.’[32] My comparison of AI planning processes leads me to concur with Ryan. Think tanks such as the Australian Strategic Policy Institute are also looking at the ADF approach to integrating AI, and conclude that as a general-purpose technology it could be applied across the ADF in a myriad ways.[33] This suggests that the integration of AI to planning may be guided by a centralised AI strategy that considers the ADF’s needs from the individual platform level up to the highest order functions such as strategic planning.
There are currently practical limitations to the role of AI in planning. Games such as Go have much more defined rules that produce definite outcomes. Gibney cautions that AlphaGo’s approach to planning may be difficult to generalise to real-world problems as ‘deep reinforcement learning remains applicable only in certain domains’.[34] This owes to limited availability and quality of data on actual military conflicts and challenges assessing the results of courses of action in the absence of simple definitions of victory and defeat. Some of the practical limitations of AI to military planning tasks might be overcome through synthetic data. Self-driving cars have been successfully trained using such a combination of real-world and synthetic data.[35] Although there continue to be significant practical obstacles to implementing an AI-enhanced planning process, there are continuous advances in the application of AI to real-world problems. These give every reason to think practical challenges will eventually be overcome.
Conclusion
It is unsurprising to conclude that AI-enhanced planning would be better applicable to the ADF than the un-augmented JMAP. By analysing one specific AI planning process in depth, however, I have been able to move past such a general conclusion and explore details that will enable or hinder this integration. The AlphaGo program understands its environment and develops and evaluates courses of action in ways that are similar to how an ADF headquarters accomplishes these tasks through the JMAP. My analysis finds no insurmountable ethical or theoretical obstacles to implementing an AI-augmented JMAP. The integration of humans in the process provides a degree of oversight that should satisfy most critics of militarised AI. Military headquarters engaged in the JMAP already incorporate non-human aids to cognition, so distributed cognition provides a good way to conceptually integrate AI. Practical challenges remain to implement AI in real conflict, an environment much more complex and less structured than the game of Go. Such challenges are being overcome in a wide variety of other applications for AI, which will no doubt enable the application of AI to military problems.
Bibliography
‘Afghanistan PowerPoint | The Fourth Revolution Blog, March 11, 2012. https://thefourthrevolution.org/wordpress/archives/2009/afghanistan-powerpoint-gr-008
American Association for Artificial Intelligence, ed. Proceedings / Sixteenth National Conference on Artificial Intelligence (AAAI-99), Eleventh Innovative Applications of Artificial Intelligence Conference (IAAI-99): July 18 - 22, 1999, Orlando, Florida. Menlo Park, Calif: AAAI Press, 1999.
Carter, Ashton B, Steinbruner, John D, Zraket, Charles A, Brookings Institution, and John F. Kennedy School of Government, eds. Managing Nuclear Operations. Washington, DC: Brookings Institution, 1987.
Commonwealth of Australia. ‘Convention On Certain Conventional Weapons (CCW) Lethal Autonomous Weapons Systems National Commentary – Australia,’ 2020. https://documents.unoda.org/wp-content/uploads/2020/08/20200820-Australia.pdf
Defense Science Board. ‘Report of the Defense Science Board Summer Study on Autonomy,’ US Department of Defense, 2016.
Department of Defence. ‘Integrated Campaigning,’ Department of Defence, 2022.
———. ‘Joint Military Appreciation Process,’ Joint Doctrine Development, 2019.
Deputy Secretary of Defense. ‘Establishment of an Algorithmic Warfare Cross-Functional Team (Project Maven).’ Department of Defense, 2017. https://www.govexec.com/media/gbc/docs/pdfs_edit/establishment_of_the_awcft_project_maven.pdf.
Engelbart, Doug. ‘Augmenting Human Intellect: A Conceptual Framework.’ Stanford Research Institute, 1963. https://www.dougengelbart.org/pubs/papers/scanned/Doug_Engelbart-AugmentingHumanIntellect.pdf.
Gibney, Elizabeth. ‘What Google’s Winning Go Algorithm Will Do next: AlphaGo’s Techniques Could Have Broad Uses, but Moving beyond Games Is a Challenge’ Nature 531, no. 7594 (March 17, 2016): 284–86.
Giddens, Anthony. The Consequences of Modernity. Stanford, Calif: Stanford University Press, 1990.
Hutchins, Edwin. Cognition in the Wild. Cambridge, Mass: MIT Press, 1995.
‘In Two Moves, AlphaGo and Lee Sedol Redefined the Future | WIRED.’ Accessed July 24, 2023. https://www.wired.com/2016/03/two-moves-alphago-lee-sedol-redefined-future/
Knorr-Cetina, K. Epistemic Cultures: How the Sciences Make Knowledge. Cambridge, Mass: Harvard University Press, 1999.
Layton, Peter. ‘The ADF could be doing much more with artificial intelligence,’ The Strategist, July 25, 2022. https://www.aspistrategist.org.au/the-adf-could-be-doing-much-more-with-artificial-intelligence/
Moy, Glenn; Shekh, Slava; Oxenham, Martin and Ellis-Steinborner, Simon ‘Recent Advances in Artificial Intelligence and Their Impact on Defence.’ DSTG, 2020. https://www.dst.defence.gov.au/sites/default/files/publications/documents/DST-Group-TR-3716_0.pdf
QIU Zhiming, LUO Rong. ‘Some Thoughts on the Application of Military Intelligence Technology in Naval Warfare,’ Air and Space Defense 2, no. 1 (January 11, 2019): 1–5.
Ryan, Mick. ‘Extending the Intellectual Edge with Artificial Intelligence, Australian Journal of Defence and Strategic Studies 1, no. 1 (2019).
Scharre, Paul. Four Battlegrounds: Power in the Age of Artificial Intelligence. First edition. New York: W.W. Norton & Company, 2023.
Silver, David; et al. ‘Mastering the Game of Go with Deep Neural Networks and Tree Search,’ Nature 529, no. 7587 (January 2016): 484–89. https://doi.org/10.1038/nature16961
Simpson, Thomas W, and Müller, Vincent C, ‘Just War and Robots’ Killings.’ The Philosophical Quarterly (1950-) 66, no. 263 (2016): 302–22.
Sparrow, Robert. ‘Killer Robots.” Journal of Applied Philosophy 24, no. 1 (January 1, 2007): 62–77.
Vaesen, Krist. “Giere’s (In)Appropriation of Distributed Cognition.” Social Epistemology 25, no. 4 (October 1, 2011): 379–91. https://doi.org/10.1080/02691728.2011.604444.
Footnotes
1 Luo Rong Qiu Zhiming, “Some Thoughts on the Application of Military Intelligence Technology in Naval Warfare,” Air and Space Defense 2, no. 1 (January 11, 2019): 1–5.
2 “In Two Moves, AlphaGo and Lee Sedol Redefined the Future | WIRED,” accessed July 24, 2023, https://www.wired.com/2016/03/two-moves-alphago-lee-sedol-redefined-future/.
3 Doug Engelbart, “Augmenting Human Intellect: A Conceptual Framework” (Stanford Research Institute, 1963), 1, https://www.dougengelbart.org/pubs/papers/scanned/Doug_Engelbart-AugmentingHumanIntellect.pdf.
4 Engelbart, ii.
5 Department of Defence, “Integrated Campaigning” (Department of Defence, 2022), 12.
6 Glenn Moy et al., “Recent Advances in Artificial Intelligence and Their Impact on Defence” (DSTG, 2020), vii, https://www.dst.defence.gov.au/sites/default/files/publications/documents/DST-Group-TR-3716_0.pdf.
7 Department of Defence, “Joint Military Appreciation Process” (Joint Doctrine Development, 2019), 2–2.
8 Department of Defence, 2–1.
9 “Afghanistan Powerpoint | The Fourth Revolution Blog,” March 11, 2012, https://thefourthrevolution.org/wordpress/archives/2009/afghanistan-powerpoint-gr-008.
10 Department of Defence, “Joint Military Appreciation Process,” 1A – 1.
11 David Silver et al., “Mastering the Game of Go with Deep Neural Networks and Tree Search,” Nature 529, no. 7587 (January 2016): 2, https://doi.org/10.1038/nature16961.
12 Silver et al., 4.
13 Defense Science Board, “Report of the Defense Science Board Summer Study on Autonomy” (Department of Defense, 2016), 14.
14 Ashton B. Carter et al., eds., Managing Nuclear Operations (Washington, D.C: Brookings Institution, 1987), 436.
15 Monte Carlo simulation uses many iterative, randomized simulations to assess the probability of an event.
16 Silver et al., “Mastering the Game of Go with Deep Neural Networks and Tree Search,” 4.
17 Paul Scharre, Four Battlegrounds: Power in the Age of Artificial Intelligence, First edition (New York: W.W. Norton & Company, 2023), 266.
18 “In Two Moves, AlphaGo and Lee Sedol Redefined the Future | WIRED.”
19 Silver et al., “Mastering the Game of Go with Deep Neural Networks and Tree Search,” 13.
20 Silver et al., 7.
21 Robert Sparrow, “Killer Robots,” Journal of Applied Philosophy 24, no. 1 (January 1, 2007): 62.
22 Thomas W. Simpson and Vincent C. Müller, “Just War and Robots’ Killings,” The Philosophical Quarterly (1950-) 66, no. 263 (2016): 305.
23 American Association for Artificial Intelligence, ed., Proceedings / Sixteenth National Conference on Artificial Intelligence (AAAI-99), Eleventh Innovative Applications of Artificial Intelligence Conference (IAAI-99): July 18 - 22, 1999, Orlando, Florida (Innovative Applications of Artificial Intelligence Conference, Menlo Park, Calif: AAAI Press, 1999), 570.
24 Commonwealth of Australia, “CONVENTION ON CERTAIN CONVENTIONAL WEAPONS (CCW) Lethal Autonomous Weapons Systems National Commentary – Australia,” 2020, 1,https://documents.unoda.org/wp-content/uploads/2020/08/20200820-Australia.pdf.
25 Commonwealth of Australia, 3.
26 Anthony Giddens, The Consequences of Modernity (Stanford, Calif: Stanford University Press, 1990).
27 K. Knorr-Cetina, Epistemic Cultures: How the Sciences Make Knowledge (Cambridge, Mass: Harvard University Press, 1999), 242.
28 Knorr-Cetina, 246.
29 Krist Vaesen, “Giere’s (In)Appropriation of Distributed Cognition,” Social Epistemology 25, no. 4 (October 1, 2011): 379, https://doi.org/10.1080/02691728.2011.604444.
30 Edwin Hutchins, Cognition in the Wild (Cambridge, Mass: MIT Press, 1995).
31 Deputy Secretary of Defense, “Establishment of an Algorithmic Warfare Cross-Functional Team (Project Maven)” (Department of Defense, 2017), 1, https://www.govexec.com/media/gbc/docs/pdfs_edit/establishment_of_the_awcft_project_maven.pdf.
32 Mick Ryan, “Extending the Intellectual Edge with Artificial Intelligence,” Australian Journal of Defence and Strategic Studies 1, no. 1 (2019): 34.
33 Peter Layton, “The ADF Could Be Doing Much More with Artificial Intelligence,” The Strategist, July 25, 2022, https://www.aspistrategist.org.au/the-adf-could-be-doing-much-more-with-artificial-intelligence/.
34 Elizabeth Gibney, “What Google’s Winning Go Algorithm Will Do next: AlphaGo’s Techniques Could Have Broad Uses, but Moving beyond Games Is a Challenge,” Nature 531, no. 7594 (March 17, 2016): 284–86.
35 Scharre, Four Battlegrounds, 23.
Comments
Start the conversation by sharing your thoughts! Please login to comment. If you don't yet have an account registration is quick and easy.